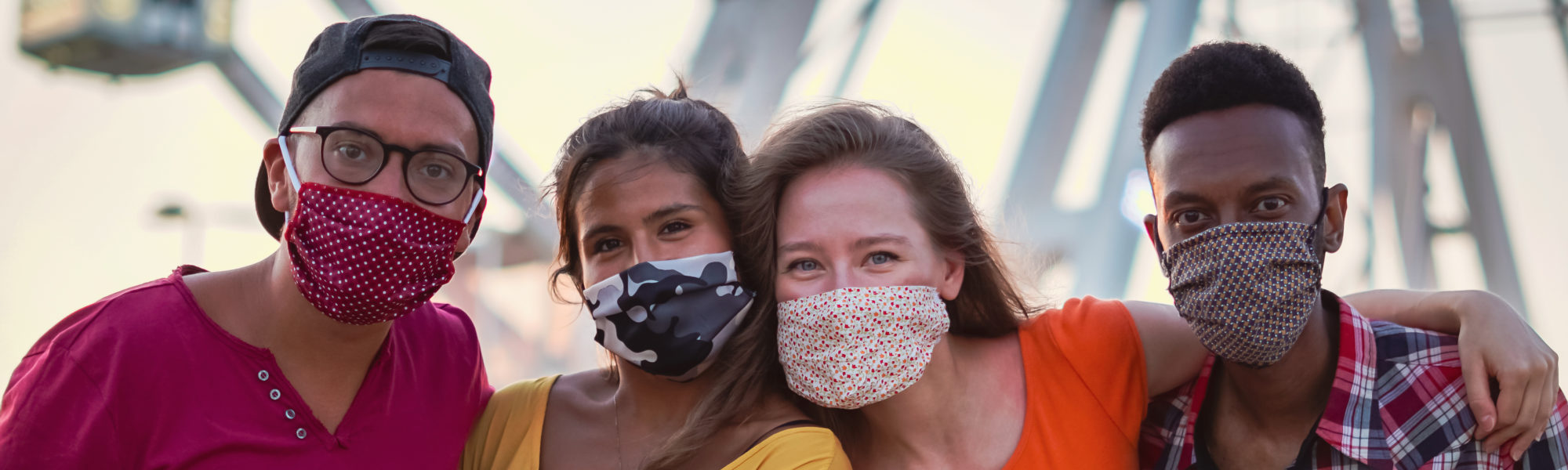
Finding and Engaging the Right Patients
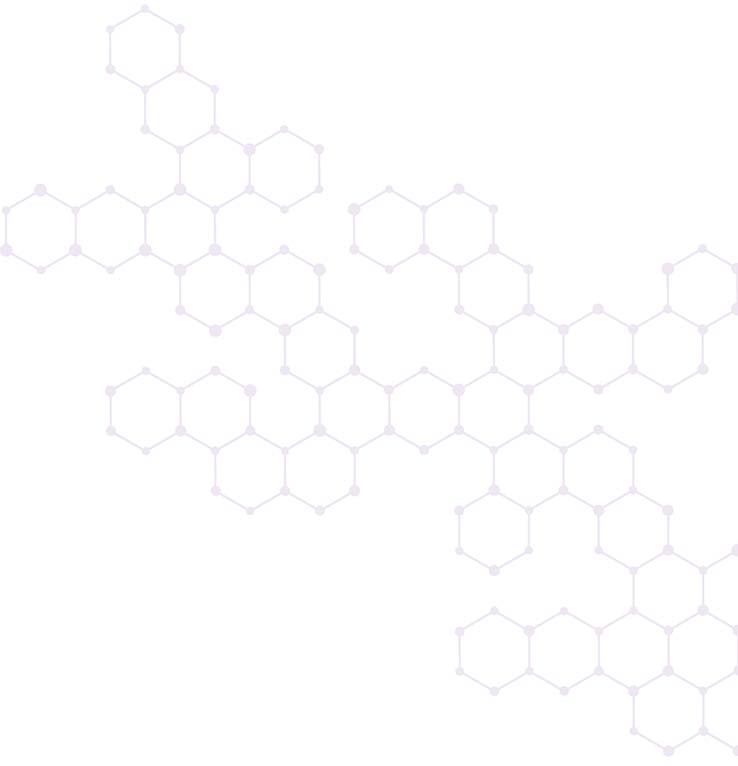
Right patient, right trial
Successful patient recruitment depends on patients not dropping out at any point in the recruitment process. Yet, there are many opportunities for that to happen. Recruitment ads may miss the mark, pre-screening technology may not work or patients may simply not consent. These variables make it hard to predict your patient enrollment rate.
Our experience and data analysis ensure greater certainty for your trial
At AES, our experience allows us to predict with confidence what patients you can expect to enroll. With permissioned data from 100 million households, we utilize these data points to identify patterns of patients most likely to enroll in a trial. This understanding helps us tailor the right message and channel based on patient preference. We track what ads patients click on, why they fail pre-screening and reasons for not consenting. In addition, we survey patients about their experiences with our patient recruitment process. We use each of these data to adjust and optimize recruitment in real time tailored to a very specific set of demographics and locations. We also scale quickly across across our 150+ research site network, bringing only the sites that will enroll your study most efficiently. Combining our data and experience, along with ensuring the right site and investigator mix, are what allow us to bring greater certainty and speed to the enrollment process.